Yang Wu
- Google Scholar
- Harbin
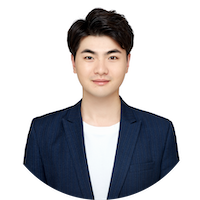
I’m a Ph.D. student in Research Center for Social Computing and Information Retrieval(SCIR), at Harbin Institute of Technology (HIT, China). I am co-advised by Prof. Bing Qin and Prof. Yanyan Zhao. My research interests include multimodal sentiment analysis and large language model.
Publications
Improving Cross-Task Generalization with Step-by-Step Instructions. SCIENCE CHINA Information Sciences
Yang Wu, Yanyan Zhao, Zhongyang Li, Bing Qin, Kai Xiong
We propose to incorporate the step-by-step instructions to help language models to decompose the tasks, which can provide the detailed and specific procedures for completing the target tasks. The step-by-step instructions are obtained automatically by prompting ChatGPT, which are further combined with the original instructions to tune language models. The extensive experiments on SUP-NATINST show that the high-quality step-by-step instructions can improve cross-task generalization across different model sizes.
Modeling Incongruity between Modalities for Multimodal Sarcasm Detection. 2021 Best Paper Award for IEEE Multimedia
Yang Wu, Yanyan Zhao, Xin Lu, Bing Qin, Yin Wu, Jian Sheng, Jinlong Li
We propose the incongruity-aware attention network (IWAN), which detects sarcasm by focusing on the word-level incongruity between modalities via a scoring mechanism. This scoring mechanism could assign larger weights to words with incongruent modalities. Experimental results demonstrate the effectiveness of our proposed IWAN model, which not only achieves the state-of-the-art performance on the MUStARD dataset but also offers the advantages of interpretability.
Sentiment Word Aware Multimodal Refinement for Multimodal Sentiment Analysis with ASR Errors. Findings of ACL 2022
Yang Wu, Yanyan Zhao, Hao Yang, Song Chen, Bing Qin, Xiaohuan Cao, Wenting Zhao
We propose the sentiment word aware multimodal refinement model (SWRM), which can dynamically refine the erroneous sentiment words by leveraging multimodal sentiment clues. We conduct extensive experiments on the real-world datasets including MOSI-Speechbrain, MOSI-IBM, and MOSI-iFlytek and the results demonstrate the effectiveness of our model, which surpasses the current state-of-the-art models on three datasets. Furthermore, our approach can be adapted for other multimodal feature fusion models easily.
A Text-Centered Shared-Private Framework via Cross-Modal Prediction for Multimodal Sentiment Analysis. Findings of ACL 2021
Yang Wu, Zijie Lin, Yanyan Zhao, Bing Qin, Li-Nan ZHU
We propose a text-centered shared-private framework (TCSP) for multimodal fusion, which consists of the cross-modal prediction and sentiment regression parts. Experiments on the MOSEI and MOSI datasets demonstrate the effectiveness of our shared-private framework, which outperforms all baselines. Furthermore, our approach provides a new way to utilize the unlabeled data for multimodal sentiment analysis.
Leveraging Multi-modal Interactions among the Intermediate Representations of Deep Transformers for Emotion Recognition. MuSe'22
Yang Wu, Zhenyu Zhang, Pai Peng, Yanyan Zhao, Bing Qin
Existing end-to-end models typically fuse the uni-modal representations in the last layers without leveraging the multi-modal interactions among the intermediate representations. In this paper, we propose the multi-modal Recurrent Intermediate-Layer Aggregation (RILA) model to explore the effectiveness of leveraging the multi-modal interactions among the intermediate representations of deep pre-trained transformers for end-to-end emotion recognition.
Locate and Combine: A Two-Stage Framework for Aspect-Category Sentiment Analysis. NLPCC 2021
Yang Wu, Zhenyu Zhang, Yanyan Zhao and Bing Qin
We propose a two-stage strategy named Locate-Combine(LC) to utilize the aspect term in a more straightforward way, which first locates the aspect term and then takes it as the bridge to find the related sentiment words. The experimental results on the public datasets show that the proposed two-stage strategy is effective, which achieves state-of-the-art performance. Furthermore, our model can output explainable intermediate results for model analysis.